The central dogma of molecular biology refers to the intricate cellular system that spans DNA replication, its transcription into RNA, and the translation of mRNA into proteins to carry out various functions. While the abundance and activity of these proteins determine cellular phenotype (note: these factors also depend on the rates of DNA transcription and RNA translation, and other mechanisms including mRNA degradation and post-translational modifications), they do not exert their function in solitude. Rather, cell behavior and phenotype depend on how these proteins interact with each other to form functional modules that carry out different critical tasks. These tasks can include basic constitutive functions like cell cycle control and metabolism, cell morphology, or even specialized functions that are unique to a cell type, such as insulin production by beta cells in pancreatic islets.
By leveraging experimentally validated (and in some cases, computationally predicted) protein-protein interactions (PPIs), we can build an entire network of PPIs that characterize a cell’s state. Generally, we expect to see different PPI networks for different cell types and states, representing different protein abundances (reflecting, for instance, the differential expression of individual protein-encoding genes or entire functional modules). Proteins that interact more frequently with one another are likely to bind each other to form protein complexes, which themselves may carry out a specific function. For instance, the popular RNA polymerase II is a complex made up of multiple proteins. At larger scales yet, PPIs may represent transient interactions such as those occurring along a pathway, like the AMPK signaling pathway or p53-mediated apoptosis, or larger functional systems like DNA damage repair. These pathways and systems can be queried using gene/protein sets from databases like the Kyoto Encyclopedia of Genes and Genomes (KEGG) and the Gene Ontology (GO). An example of a PPI sub-network is visualized below:
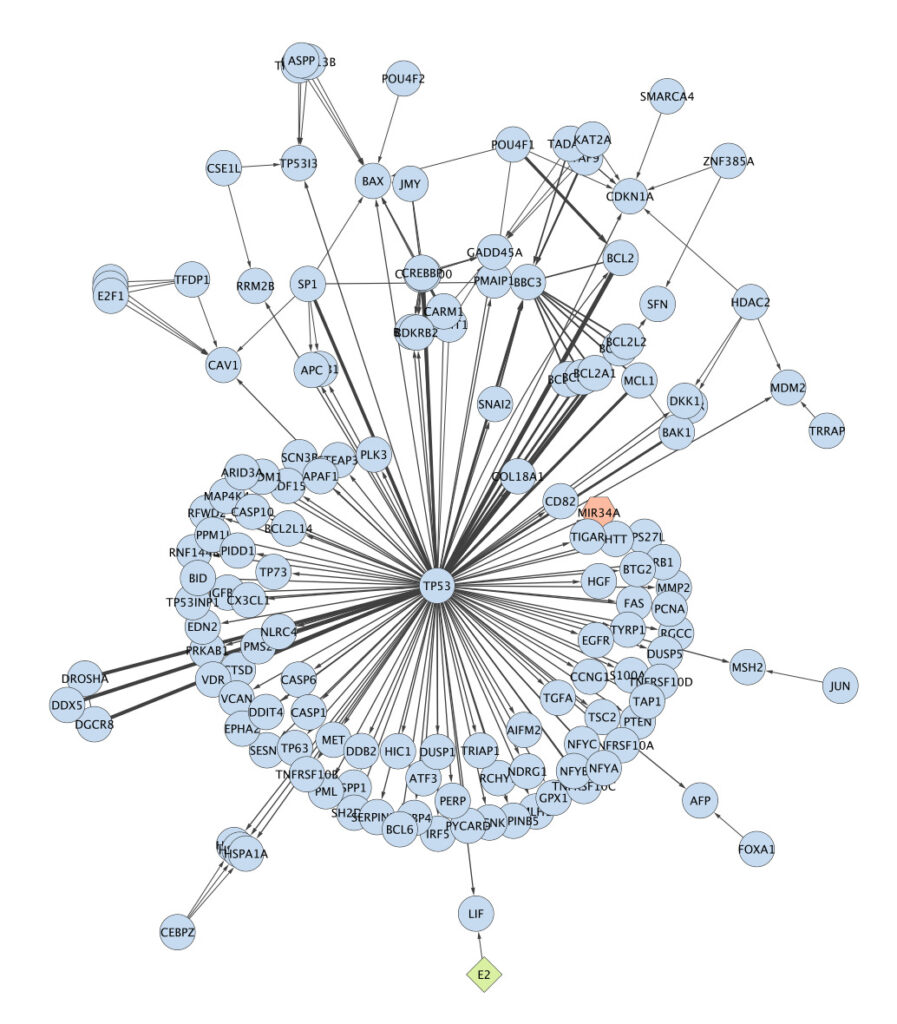
The analysis of PPI networks is critical to understanding how genetic abnormalities lead to dysfunctional cellular pathways and systems, contributing to disease development. For example, the misfolding of proteins and aberrant interactions, as observed in neurodegenerative diseases like Alzheimer’s, exemplify this. Amyloid-beta oligomerization, ultimately an aberrant PPI, is a key factor in Alzheimer’s disease pathology. In cancer, a mutated TP53 gene serves to accelerate cancer by inhibiting its tumor suppressive functions, dysregulating cell cycle control and apoptosis by impacting the P53 protein’s interactions with numerous other proteins. It should be clear now that PPIs are critically important for our understanding of the cell; a disease-causing mutation produces a disease phenotype by its impact on protein function, or the functional module to which that protein belongs, not by its own virtue.
A brief overview of network analysis
PPI networks can be represented as a collection of nodes and edges, where edges may be binary (1 – protein A interacts with protein B, 0 – else) or weighted by confidence of the PPI. We can analyze the statistics of this network (also referred to as a graph) and extract interesting information from it. For example, PPI networks tend to be approximately scale-free, meaning that only a few proteins (hubs) have numerous connections, whereas other proteins are only sparsely connected, providing stability and robustness to the network. The TP53 protein in human cells is a classic example of a hub protein, interacting with several other proteins to regulate cell cycle and apoptosis. Such hub proteins are critical for network integrity, where their disruption can lead to significant cellular dysfunctions, as seen in the case of TP53 or BRCA1 in breast cancer, where mutations disrupt its role in DNA repair. PPI networks are also very modular, which ensures functional specificity and efficiency. For instance, in signal transduction pathways, modular interactions allow for the precise control and amplification of signal. Thus, just be analyzing the network connectivity of proteins, we can make inferences about how mutations would impact cell function or specific subcellular systems.
Applications in bioinformatics and medicine
The mapping of PPI networks has been pivotal in understanding complex diseases. In cancer research, the elucidation of PPI networks involving oncogenes and tumor suppressors has opened new avenues for targeted therapies. For example, the interaction network of the BCR-ABL proteins has been crucial in developing targeted therapies for chronic myeloid leukemia. PPI networks also guide the identification of novel drug targets. Kinase inhibitors in cancer therapy, such as Imatinib, were developed based on our understanding of kinase interaction networks. To this end, platforms like STRING and BioGRID have further enabled researchers to visualize and analyze PPI networks, facilitating the identification of potential biomarkers and therapeutic targets. We can also leverage PPI networks to model and simulate cellular processes, thereby providing insights into systemic functions and interactions. The integration of PPI data with genomic information has been instrumental in annotating gene functions and understanding the structure of gene regulation networks.
Conclusion
Thus, PPI networks serve as a linchpin in the understanding of how molecular dynamics govern our lives at the cellular level. The comprehensive study of these networks characterizes the interplay of proteins within cells and unravels the low-level etiologies of health and disease. As we continue to explore and decode PPI networks, their potential to transform our approach to medicine, drug development, and our overall comprehension of biological systems remains immense. The future of biomedical research and therapeutic innovation is intricately linked to our ability to leverage and manipulate these complex networks.
Wow! Amazing content!